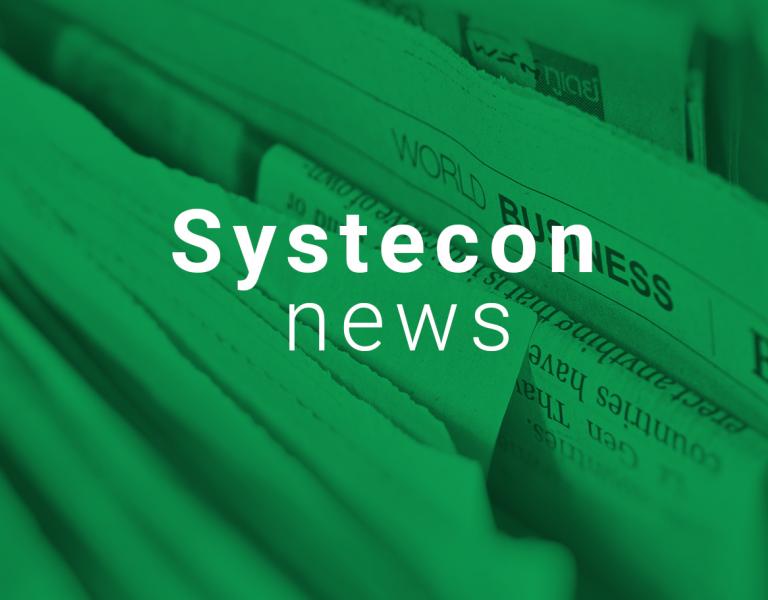
The art of efficient modeling
Throughout history, people have tried to understand the world and its phenomena by describing it in the form of models. A good model makes it easier to make the right decisions and generates significant benefits, while a poor model gives incorrect predictions and leads to poor decisions.
Throughout history, people have tried to understand the world and its phenomena by describing it in the form of models. A good model makes it easier to make the right decisions and generates significant benefits, while a poor model gives incorrect predictions and leads to poor decisions.
For example, we have enjoyed the benefits of being able to predict the course of the year with the solar calendar. But man has also for centuries been confused by astrological calendars, even though they both use the motion of the celestial bodies as their foundation. As with other models it is also important to model wisely and build the model efficiently when modeling with Opus Suite in order to achieve a sound basis for decision-making.
In the era of big data the need for good and efficient models is even more important. Today, it is easy to collect, save and process very large amounts of data, but it is still difficult to use this data in the right way and transform it into relevant information for decision-making. To succeed and reap the full benefits of the data plenty, efficient modeling is required.
Efficient modeling means to create models that quickly, accurately and effectively can provide the answers needed. For a model to be considered efficient it must also easily be able to adapt to changes in prerequisites, questions and data.
The model that forms the backbone of Opus Suite is comprehensive and provides a high degree of flexibility to the user. Consequently, the model provides plenty of opportunities to reflect scenarios and phases that occurs in the operations and maintenance of technical systems. However, with this level of flexibility, there is a risk of losing focus on building an efficient model and to instead try to put in as much of the available information as possible. It is, of course, important to get many different aspects reflected in the model, but not more than what is possible to understand and where it is possible to explain the effects of the input on the results. The focus must always be on the task in hand. To be able to do this a number of practical tips to keep in mind are presented.
Focusing on the issue
Determining what issue that you want to address is the critical first step, which must be kept in mind throughout the modeling. It is easy to lose this focus if the modeling is controlled by what data that is available or if there’s excessive reliance on the individual's perception of what is important to take into account, instead of the underlying question to be answered. During the modeling the question often changes with new input and new insights, and then it is important that the model is adjusted and flexible enough to support this.
Start early
To succeed in getting a as complete and clear result as possible from the model, it is important to start early. In addition to getting answers, the modeling will also lead to a deeper understanding. The sooner the work begins the more opportunities there are to understand the model and its results, as well as supplement the model where data is missing or lacking in quality. By starting early you have more opportunities to understand the issue better and gradually adjust the model to the real needs.
Limit the complexity
To be sure that the model really answers the current question, it is important to limit complexity. A too large and complex model makes it difficult to understand the causal links between input and results.
Working iteratively
To constantly keep control over the model, the model development should be considered as a continuous iterative process. It is wrong to believe that it is possible to build a complete model and run it once to get good results. Only through iterations are continuous improvements and increased understanding achieved.
Gradual refinement
An extensive and time-consuming task in the work with producing decision support is to collect and process data to a degree of sufficient quality. The focus of this work should be on the data that the model and the issue require and be sufficient rather than complete. It is better to start modeling with rough estimates than to try and create a complete data set of perfect quality. Otherwise, there is an immediate risk in getting stuck in the data collection process. To achieve good results, the input should be gradually refined and the model should be used to assess where more detailed and accurate data is most important. The reality is that the model and its results are the best tools to quickly increase the quality of input data and help focus on where there is a need for more detail.
Question the model and results
Throughout the analysis, both the model and the results should be questioned. By critically examining the results and trying to explain why they look the way they do, or by monitoring how a change in input data impacts on the results and trying to explain it, one is often surprised by the many insights that can result and how intuitive expectations may not always be correct. To be able to question the model effectively, you must also, as an analysist, challenge yourself. The basic model of Opus Suite is comprehensive and the relations between the different parts is complex and it is therefore important to always try to learn more of how the model behaves even for the experienced modeler.
Verify model
Finally, the model should be verified by using key figures in the results that can be checked against actual, calculated or estimated data. There are many different ways to do this. All models differ from each other and it is also the best way to verify them. Examples on key figures to use could be to ensure that the number of maintenance actions that the model predicts are reasonable, or that the proposed inventory levels don’t differ greatly from existing or expected stock. Each model should be measured against their own verification criteria.
Summary
A major and challenging task is to build efficient models of complex and extensive scenarios, especially with the increasing amount of data available. By keeping the focus on the questions to be answered, being adaptable through an iterative work and constantly maintaining control over how the model is developed, it is possible to quickly and effectively generate decision support that is clear, accurate and provides a significant benefit. And by sticking to the points suggested above, it is easier to achieve an efficient model, making it easier to navigate and achieve better results more quickly.